Note
Go to the end to download the full example code.
Histogram 3D
This 3D histogram class allows efficient updating of histograms, plotting and saving as HDF5.
import geobipy
from geobipy import StatArray
from geobipy import Histogram
import matplotlib.pyplot as plt
from geobipy import RectilinearMesh3D
import numpy as np
Create some histogram bins in x and y
x = StatArray(np.linspace(-4.0, 4.0, 11), 'Variable 1')
y = StatArray(np.linspace(-4.0, 4.0, 21), 'Variable 2')
z = StatArray(np.linspace(-4.0, 4.0, 31), 'Variable 3')
mesh = RectilinearMesh3D(x_edges=x, y_edges=y, z_edges=z)
Instantiate
H = Histogram(mesh=mesh)
Generate some random numbers
a = np.random.randn(100000)
b = np.random.randn(100000)
c = np.random.randn(100000)
# x = np.asarray([a, b, c])
Update the histogram counts
H.update(a, b, c)
plt.figure()
plt.suptitle("Slice half way along each dimension")
for axis in range(3):
plt.subplot(1, 3, axis+1)
s = [5 if i == axis else np.s_[:] for i in range(3)]
_ = H[tuple(s)].pcolor(cmap='gray_r')
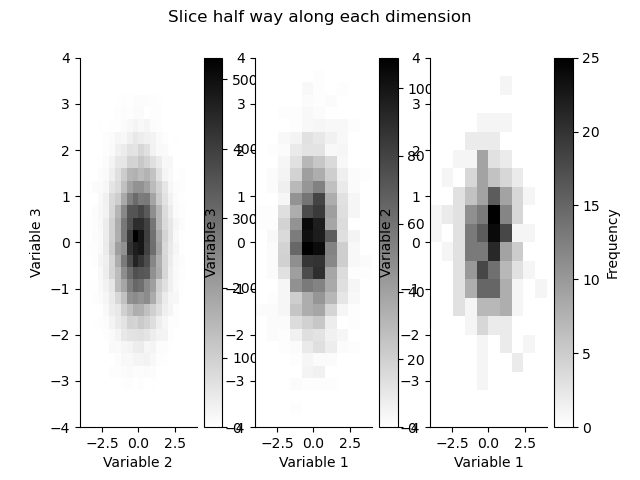
Generate marginal histograms along an axis
plt.figure()
plt.suptitle("Marginals along each axis")
for axis in range(3):
plt.subplot(1, 3, axis+1)
_ = H.marginalize(axis=axis).plot()
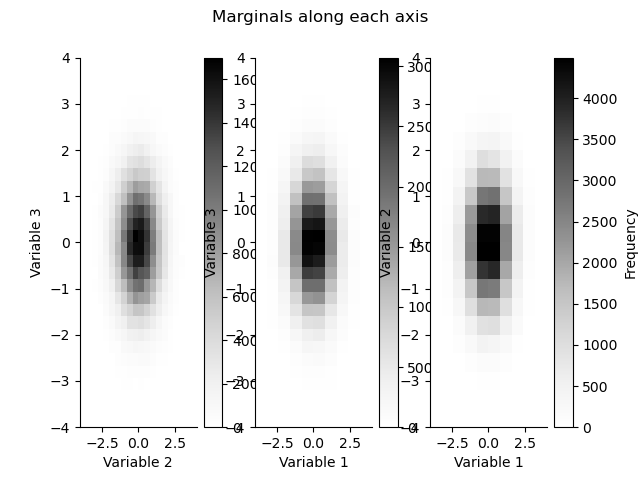
Take the mean estimate from the histogram
plt.figure()
plt.suptitle("Mean along each axis")
for axis in range(3):
plt.subplot(1, 3, axis+1)
_ = H.mean(axis=axis).pcolor()
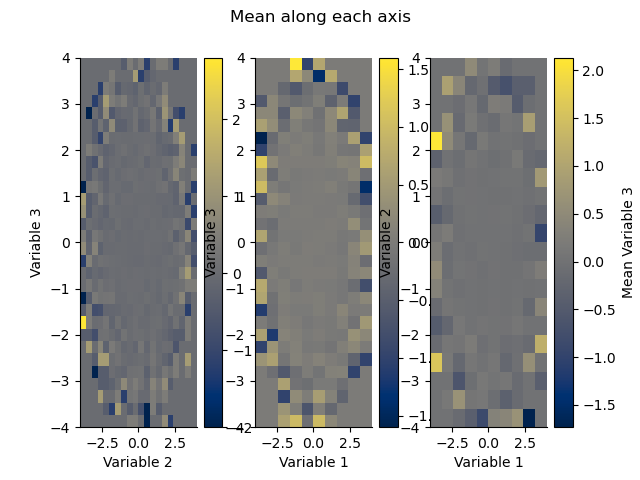
Take the median estimate from the histogram
plt.figure()
plt.suptitle("Median along each axis")
for axis in range(3):
plt.subplot(1, 3, axis+1)
_ = H.median(axis=axis).pcolor()
# #%%
# # We can map the credible range to an opacity or transparency
# H.opacity()
# H.transparency()
H.animate(0, 'test.mp4')
H.to_vtk('h3d.vtk')
# Create some histogram bins in x and y
xx, yy = np.meshgrid(mesh.z.centres, mesh.y.centres)
x_re = StatArray(np.sin(np.sqrt(xx ** 2.0 + yy ** 2.0)), "x_re")
xx, yy = np.meshgrid(mesh.z.centres, mesh.x.centres)
y_re = StatArray(np.sin(np.sqrt(xx ** 2.0 + yy ** 2.0)), "y_re")
xx, yy = np.meshgrid(mesh.y.centres, mesh.x.centres)
z_re = StatArray(np.sin(np.sqrt(xx ** 2.0 + yy ** 2.0)), "z_re")
mesh = RectilinearMesh3D(x_edges=x, x_relative_to=x_re, y_edges=y, y_relative_to=y_re, z_edges=z, z_relative_to=z_re)
Instantiate
H = Histogram(mesh=mesh)
Generate some random numbers
a = np.random.randn(100000)
b = np.random.randn(100000)
c = np.random.randn(100000)
# x = np.asarray([a, b, c])
Update the histogram counts
H.update(a, b, c)
plt.figure()
plt.suptitle("Slice half way along each dimension")
for axis in range(3):
plt.subplot(1, 3, axis+1)
s = [5 if i == axis else np.s_[:] for i in range(3)]
_ = H[tuple(s)].pcolor(cmap='gray_r')
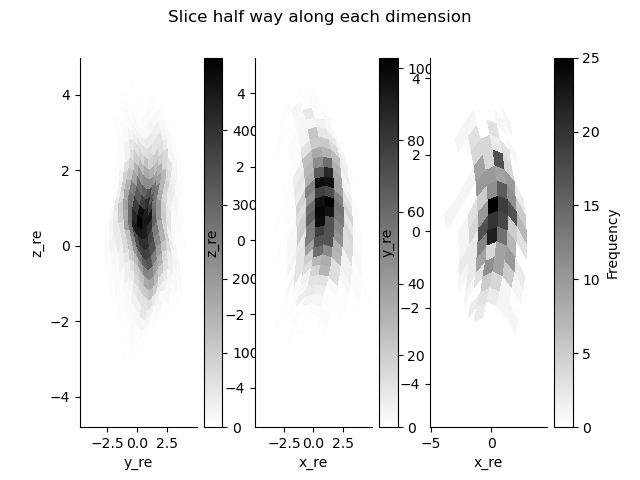
Generate marginal histograms along an axis
plt.figure()
plt.suptitle("Marginals along each axis")
for axis in range(3):
plt.subplot(1, 3, axis+1)
_ = H.marginalize(axis=axis).plot()
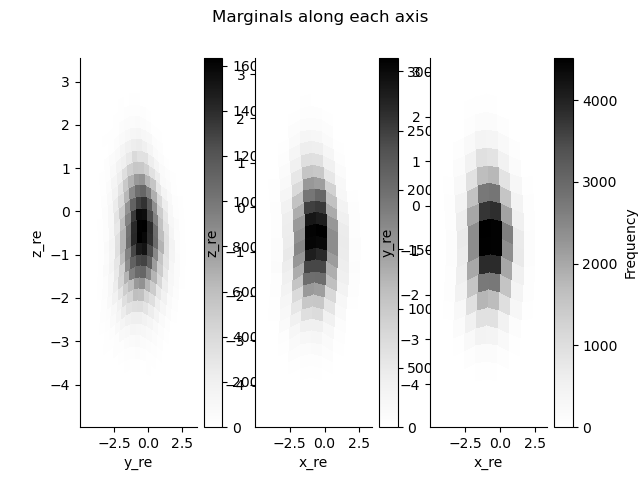
Take the mean estimate from the histogram
plt.figure()
plt.suptitle("Mean along each axis")
for axis in range(3):
plt.subplot(1, 3, axis+1)
_ = H.mean(axis=axis).pcolor()
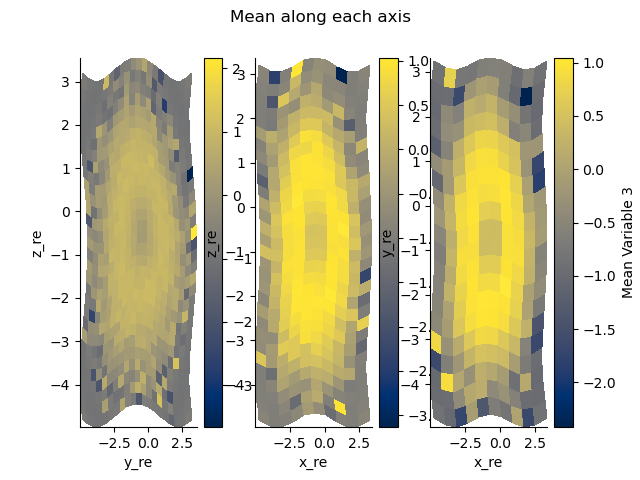
Take the median estimate from the histogram
plt.figure()
plt.suptitle("Median along each axis")
for axis in range(3):
plt.subplot(1, 3, axis+1)
_ = H.median(axis=axis).pcolor()
# #%%
# # We can map the credible range to an opacity or transparency
# H.opacity()
# H.transparency()
H.animate(0, 'test.mp4')
plt.show()
# H.to_vtk('h3d.vtk')
Total running time of the script: (0 minutes 4.437 seconds)